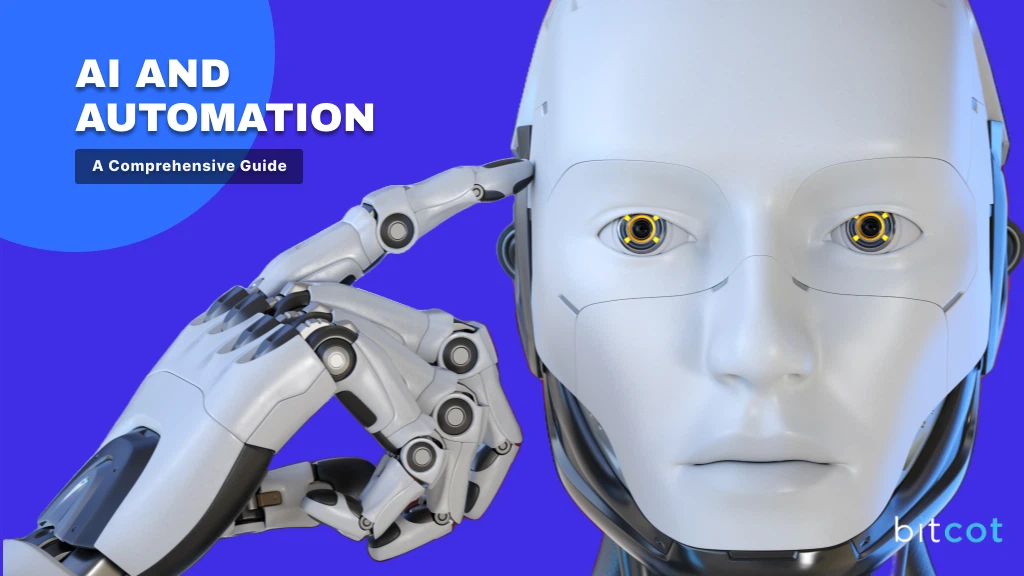
In 2025, all we see and hear is a new chat tool, a new plugin, or a new software that works in AI. Just type your query, and an answer will pop up within seconds has become the golden standard in digital interactions right now.
AI and Automation aren’t new, but recent breakthroughs in technology are pushing the boundaries of what machines can accomplish. Forward-looking businesses are finding new ways to establish more efficient processes and implement innovative improvements for years to come.
Beyond regular industrial automation and sophisticated robots, we’re seeing new generations of more advanced systems like self-driving cars on roads and automated check-outs in grocery stores. Such incredible advancements are making headlines, many involving capabilities that are way beyond what humans can do.
This article explores how you can effectively employ AI for automation and make (once unimaginable) progress on some of your most difficult business challenges.
What Are Artificial Intelligence and Automation?
Artificial Intelligence (AI) is basically computers trying to show intelligence as close to humans as possible, including learning, reasoning, and self-correction.
AI consists of various subfields like Machine Learning (ML), Natural Language Processing (NLP), Computer Vision, and Robotics.
These AI technologies aim to create systems capable of performing tasks that require human intelligence, including understanding natural language, recognizing patterns in data, making decisions, and interacting with the environment in ways that mimic human cognition.
For example, chatting with an AI virtual assistant could be an experience similar to having a conversation with a human.
Another example is a recommendation system suggesting what a user would want to buy if they’re interested in item “X.” This can’t be just coded or logically apprehended with lines of code.
Such a type of analysis requires a learning mechanism capable of adapting to changes in data and identifying patterns to make decisions.
In 2024, AI is much more complex and processes larger amounts of data.
Today’s examples of AI include advanced NLP models like GPT-4, diagnostic systems like IBM Watson, collaborative manufacturing robots, neuromorphic chips, etc.
According to a 2023 IBM Global AI Adoption Index report, about 42% of enterprise-scale organizations surveyed actively use AI in their businesses. This adoption reflects the growing recognition of AI’s transformative power.
Now let’s move on to Automation, which is the use of technology and systems to perform tasks or processes that were previously carried out by humans.
These tasks involve applications like Business Process Automation (BPA), IT Automation, network automation, integration between systems, industrial automation like robotics, and consumer electronics.
The goal of Automation is to optimize operations and reduce errors by replacing manual labor with automated processes.
Businesses across the world are fascinated with the idea of automation because it promises improved operational efficiency, optimized processes, and considerable cost savings.
Automation can range from simple repetitive tasks like data entry or document processing to complex workflows involving multiple steps and decision points.
Task Automation takes repetitive, simple tasks and automates them. This helps eliminate errors and free up people’s time to do higher-value, more meaningful work. A prime example of Task Automation is Robotic Process Automation (RPA).
Process Automation takes more complex, multi-step processes by integrating various systems and automating them to boost efficiency and productivity. Process Mining and Business Process Management (BPM) are prime examples of Process Automation.
What Are the Differences Between AI and Automation?
AI and Automation serve common purposes: to help organizations operate smarter and more efficiently. However, they’re both completely distinct concepts. To begin, let’s see quick definitions of both.
- Automation is about setting up robots that follow a set of pre-defined rules.
- AI is about setting up robots that make their own decisions.
Now, let’s take a closer look at these terms to get a better idea of their differences.
Automation is robots following orders. Humans define the rules and the robots perform them. That’s the essence of automation.
The point of this is to free us from monotonous tasks that are tiresome and boring. When we humans perform repetitive tasks, we tend to not only get bored but also make mistakes. Robots don’t, and they can also carry out these tasks better and faster.
Where Automation is the robot’s arm, AI is the robot’s brain.
The goal of AI isn’t to perform mundane tasks like Automation. Instead, it’s designed to mimic humans at a more intellectual level, capable of performing tasks through observation of patterns and past outcomes.
To put it another way, they’re designed to learn and act on what they have learned, instead of merely taking orders.
Here’s a table comparing the key differences between Automation and Artificial Intelligence.
Parameter | Automation | Artificial Intelligence |
Definition | Performing repetitive tasks by following predefined rules. | The ability of machines to learn from experience, adjust to new inputs, and perform human-like tasks. |
Process | Rules are predefined by humans for machines to follow. | Machines learn on their own from large amounts of data to solve complex problems. |
Examples | Assembly lines, data entry bots, and rule-based loan approval. | Siri, self-driving cars, and algorithms that learn from user behavior. |
Role of Humans | Define processes and rules for tasks. | Define objectives and problems. |
Scope of Tasks | Structured, repetitive tasks with defined steps. | Unstructured problems that require reasoning and cognitive abilities. |
Intelligence Level | Narrow, can only do predefined tasks, with limited AI capabilities. | Can exhibit general intelligence and learn new tasks. |
Requirement | Well-defined processes. | Large amounts of data for machine learning algorithms. |
How Do AI and Automation Work Together?
Now that we’ve explored the main differences between Automation and Artificial Intelligence, let’s take a closer look at how they’re similar and how they work together.
A common characteristic of Automation and AI is that they both run on data. Where automated machines collect data, AI systems process it.
Automation and AI can work together to achieve a lot more than either could do on its own. By using them together, you can not only gather and transfer data, you can also interpret that data, and base automated actions on those insights.
In 2024, automation’s capabilities have expanded to include sophisticated decision-making abilities, facilitated by advanced AI integration.
That team-up is where you get the Cognitive Automation which is commonly called Intelligent Automation (IA). This reverse acronym of AI empowers robots to take the general outlines laid out by humans and develop their own pathways to attain the goal.
This means that machines can continually “learn”, improving decision-making and actions by leveraging data from previously encountered and analyzed situations.
An Example of AI and Automation Working Together
Imagine a company that has a customer service center.
Every day, thousands of emails are received by them – so many that they cannot respond to those emails within a 24-hour time span with their existing resources.
Without hiring more people, the management wants to find a way for customers to get their issues resolved immediately by email or by getting in touch with a customer service rep.
To make this happen, the company automates the email classification process. The automation bot identifies keywords in the emails, categorizes them into different folders, and then assigns them to the right rep.
This speeds up the process, but it still doesn’t enable customers to receive an immediate response to their inquiries. For this, AI is needed.
Automation AI, more specifically Natural Language Processing (NLP), can be used to interpret the intent of the request in the email. Based on that understanding, the AI robot can then send out a response right away that immediately resolves the customer’s problem.
That’s the power of Artificial Intelligence Automation.
What are the Use Cases of Intelligent Automation?
Cognitive Decision-Making
RPA is good at executing predefined rules and instructions, but it lacks the ability to make complex decisions based on unstructured data or situations where the rules aren’t clearly defined. For tasks requiring cognitive decision-making abilities like fraud detection, risk assessment, and medical diagnosis, IA is needed.
For example, when assessing loan applications, RPA can efficiently process and validate applicant data against predefined criteria like credit score and income level. However, when it comes to making complex decisions about loan approvals, especially for unconventional cases or those with vague financial histories, RPA falls short.
Determining the creditworthiness of a self-employed individual with irregular income streams requires nuanced judgment beyond the scope of predefined rules, a capability offered by IA.
IA can analyze diverse data sources including transaction histories and market trends, to make informed decisions on loan approvals, considering contextual factors,factors and mitigating risks more effectively.
As new loan decisions are made and their outcomes are observed, the IA system receives feedback on the accuracy of its predictions. It continuously learns from this feedback, updating its models and refining its decision-making criteria to adapt to changing conditions and improve performance over time.
Natural Language Understanding
RPA tools typically struggle with understanding and processing human language. So use cases like customer support chatbots, sentiment analysis, and language translation require IA solutions that can comprehend and generate human-like responses.
For example, an RPA-based chatbot might be programmed to recognize keywords and phrases to provide basic information or route queries to appropriate departments. However, it might struggle with understanding context or accurately interpreting the nuances of customer inquiries.
In contrast, an IA chatbot can understand the intent behind customer messages, analyze sentiment, and provide relevant responses in a conversational manner.
If a customer is on the verge of a meltdown about a delayed delivery, the IA chatbot can empathize and offer assistance.
As it interacts with more customers, it continuously learns and improves its ability to comprehend and respond to a wide range of language variations and contexts.
Complex Data Analysis
While RPA can handle basic data entry and manipulation tasks, it may not be suitable for analyzing large volumes of complex or unstructured data. So use cases like predictive analytics and anomaly detection require IA solutions with advanced data processing and ML capabilities.
For example, an RPA solution in a manufacturing setting might handle basic data entry tasks related to equipment maintenance schedules like inputting maintenance logs into a database. However, it lacks the capability to analyze large volumes of sensor data from machines or predict equipment failures proactively.
An IA solution can continuously monitor sensor data from manufacturing equipment, identify patterns indicative of potential failures, and predict when maintenance is required.
By analyzing temperature, vibration, and other sensor readings, the IA system can forecast when a machine is likely to malfunction, allowing maintenance to be scheduled proactively to avoid costly downtime.
As the IA system learns from historical data and feedback, it becomes increasingly accurate in its predictions, optimizing maintenance schedules and improving overall equipment reliability.
Creative and Innovative Tasks
Tasks that require human creativity, intuition, and problem-solving skills are beyond the scope of RPA. So use cases like product innovation, design thinking, and strategic planning can benefit from IA.
For example, IA enhances product innovation in the automotive industry by leveraging advanced technologies like ML and simulation.
IA systems analyze vast data sets to identify emerging trends and consumer preferences, help in virtual simulations to optimize car designs, and optimize prototyping and testing processes.
These insights serve as valuable inputs for creative brainstorming sessions, guiding designers and engineers toward innovative solutions that align with market demands.
By facilitating real-time collaboration and providing insights for creative decision-making, IA accelerates innovation cycles, improves design quality, and ensures automotive companies remain competitive.
What are the Benefits of Intelligent Automation?
With the automation of tasks through AI, organizations can intelligently scale automations, enhance consistency within their workflows, and maximize returns.
Improved Decision-Making
While RPA can automate time-consuming tasks, IA goes further by leveraging advanced analytics and ML to provide insights and recommendations that support better decision-making. This involves analyzing complex datasets and identifying patterns to make strategic decisions more than people ever could.
Enhanced Customer Experience
While RPA can automate certain aspects of customer interactions, IA offers personalized and responsive customer experiences. By integrating with AI-powered chatbots, sentiment analysis tools, and other advanced technologies, it can deliver tailored recommendations, faster response times, and higher levels of customer satisfaction.
Enhanced Scalability
While RPA can automate specific tasks within predefined workflows, IA offers broader scalability by integrating with other technologies like AI and ML. This allows organizations to automate more complex processes and handle larger volumes of work without the need for additional resources.
Innovation and Competitive Advantage
While RPA optimizes existing processes, IA fosters innovation by freeing up employees to focus on more challenging and creative aspects of their work. This enables organizations to free their team to work on things that need a human touch, innovate, develop new products or services, and maintain a competitive edge in the market.
A Real-world Example of Automation and AI
Let’s take a quick look at a case study from Bitcot that will show you how Automation and AI are changing the way things work in the real world and making a big difference in how businesses operate.
This project of ours, LAS, is within Microsoft’s Power Automate framework. Here, AI plays a crucial role in optimizing data extraction processes.
When an invoice arrives in the form of an email attachment in PDF format, it triggers a series of automated actions. Initially, AI is employed to read and extract relevant information from the text within the invoice. This includes predefined fields such as purchase order numbers, purchase IDs, dates, and vendor names.
Upon extraction, the data is organized and managed using rules set within the automation system. For instance, specific folders are designated for invoices from different suppliers. Using predetermined criteria, the system automatically sorts incoming invoices into their respective folders.
Once the invoice is routed to the appropriate folder, further automation processes are initiated.
At this stage, the AI-driven automation triggers a flow that involves transferring the PDF to a designated location such as OneDrive. Here, the PDF is split into individual pages using a third-party tool, Muhimbi. Each page undergoes text extraction, facilitated by AI, to retrieve essential data points.
These data are then collated and managed within an Excel sheet, where they may undergo additional processing or be transferred to other systems via an API tool – the VAS database.
Throughout this entire process, from the initial receipt of the invoice email to the final data management stage, automation and AI work together to enhance efficiency and accuracy.
AI is essential alongside RPA because it can understand messy data better. While RPA is good at doing tasks and workflows that follow clear rules, it can’t understand and extract meaning from unstructured data like the text within PDF invoices.
AI is great at making sense of this messy data, adding an extra layer of intelligence. It uses NLP to read the text in invoices and figure out important details.
This intelligence makes the automation process more smoother, accurate, and adaptable to different types of invoices.
Our case study demonstrates how AI-powered automation can optimize complex tasks, improve data-handling processes, and ultimately drive operational effectiveness within an organization.
How Does Intelligent Automation Software Work?
Intelligent Automation software combines various technologies, including Automation, RPA, BPM, AI, ML, DL, and advanced analytics, to automate tasks and processes in a smarter and more sophisticated manner.
It covers the entire automation journey – discovery, automation, optimization – automating any front-office or back-office business process and coordinating work across combined human-bot teams.
Here’s how it works:
Process Understanding
The software begins by understanding the tasks and processes it aims to automate. This involves mapping out the steps involved, identifying inputs and outputs, and recognizing decision points within the process.
Data Ingestion
The software collects and ingests data from various sources, including structured data from databases, spreadsheets, and forms, as well as unstructured data from documents, emails, and other text sources.
Data Processing and Analysis
Using AI and ML algorithms, the software processes and analyzes the data to identify patterns, correlations, and insights. This may involve tasks like data classification, sentiment analysis, and predictive modeling.
Decision Making
Based on the analysis, the software makes decisions and takes actions autonomously or with minimal human intervention, including determining the next steps in a process, applying business rules, or triggering specific actions based on predefined criteria.
Task Automation
The software automates tasks and processes by mimicking human actions. This can involve interacting with applications, entering data, retrieving information, performing calculations, and executing repetitive tasks with high accuracy and efficiency.
Learning and Optimization
The software continuously learns from its interactions and experiences. Through ML, it can improve its performance over time, adapt to changing conditions, and optimize processes for greater efficiency and effectiveness.
Integration and Orchestration
The software integrates with existing systems, applications, and technologies within the organization’s IT infrastructure. It orchestrates workflows and coordinates activities across different systems to ensure end-to-end automation.
Getting Started with Intelligent Automation
Understand the Basics
Start by familiarizing yourself with the fundamentals of AI in Automation. Recognize how it differs from traditional RPA and its potential to transform various aspects of your organization. This paves the way for strategic deployment and leveraging its broader capabilities for organizational growth and efficiency.
Assess Your Needs
Evaluate your organization’s processes and identify areas where IA can make a significant impact. Look for tasks that keep coming back, take forever to complete, have a tendency for errors, and deal with large amounts of data as these are prime candidates for automation.
Set Clear Objectives
Define clear objectives and goals for your IA implementation. Whether it’s improving decision-making, enhancing customer experience, or optimizing compliance processes, having a clear vision will guide your efforts effectively. It provides a roadmap for IA initiatives, facilitating measurable outcomes that drive success.
Collect the Necessary Data
AI systems need data to learn patterns, correlations, and relationships within the data and make predictions, classify information, etc. The more quality data you can get, the better the performance of the AI model. More high-quality data ensures that the model learns from a wide range of reliable and representative examples, ensuring accuracy.
Select the Right Tools
Choose the appropriate IA tools and technologies that align with your objectives. This may include AI-powered analytics platforms, ML algorithms, chatbots, and workflow automation software. Ensure that the selected tools integrate smoothly with your existing systems.
Build a Strong Foundation
Invest in developing a solid infrastructure to support your IA initiatives. This includes establishing data governance practices, ensuring data quality, and providing adequate training to your team members. By prioritizing these aspects, you can enable efficient automation and effective utilization of IA technologies across the organization.
Start Small
Don’t try to automate everything at once. Start by automating a few tasks or processes internally to adapt to the automation environment and then prepare to serve customers and employees. You can start with small-scale pilot projects to test the waters and demonstrate the value of automation.
Automate Right
Not every repetitive task is worth automating so make sure you automate the right things. Go for the tasks that add significant value and consume a substantial amount of time. By focusing on these types of tasks, you can save time, cut down on errors, and make your processes more scalable.
Iterate and Scale
Regularly monitor and evaluate the performance of your IA initiatives. Identify areas for improvement and iterate on your processes to optimize efficiency and effectiveness. As you gain confidence and experience, gradually scale up your automation efforts across the organization.
Seek Expert Guidance
Consider partnering with IA experts to accelerate your journey and ensure successful implementation. Use their expertise to navigate challenges, mitigate risks, and maximize the benefits of IA. If you’re ready to take the next step, feel free to reach out to our team here at Bitcot, we’ll be glad to help by offering guidance and comprehensive services.
How to Automate More with AI
Before Gen AI’s boom, which started in late 2022, AI had already played a role in automation software. But now, the emergence of Gen AI is fueling more productivity gains, empowering developers to build internal chatbots, summarize documents, email response generators, and more, faster.
A foundation model, like a pre-trained language model like GPT (Generative Pre-trained Transformer), can be used to enhance the IA software’s capabilities in the following ways:
- Generate new text that’s invaluable for businesses needing to generate large volumes of content for marketing, customer support, or product development purposes. For example, AI-generated text can be used to create product descriptions, blog posts, or social media posts, saving time and resources.
- Simulate and model complex systems, enabling businesses to optimize processes, predict outcomes, and make informed decisions. For example, AI-generated simulations can help manufacturers optimize production lines, retailers forecast demand, or model risk factors.
- Automate complex tasks that traditionally require human intervention, including content creation, data synthesis, or even software development. By automating these tasks, businesses can increase efficiency, reduce errors, and free up human resources for more strategic initiatives.
- Effectively engage users in natural conversations using deep learning architectures like Transformers that have been trained on vast amounts of data and user interactions. This allows chatbots to understand context and generate human-like text with greater accuracy, fluency, and creativity.
- Facilitate product innovation by generating new ideas, concepts, or prototypes. For example, AI-driven ideation platforms can assist R&D teams in brainstorming new product features or designs, leading to the development of innovative offerings that meet market demands.
Key Takeaways
In this article, we’ve learned what is AI Automation. To sum up, the technology space’s new power couple combines the cognitive “thinking” capabilities of AI with the “task performing” capabilities of RPA to optimize business processes.
With AI and its allied technologies, RPA bots can mimic a broad spectrum of the decision making and problem solving that people do, expanding the scope of work that you can automate.
And now, with Gen AI making its emergence, the automation possibilities just got a whole lot wider.
According to Automation Anywhere’s 2023 Now & Next report, 78% of companies say productivity gains are a desired impact of Intelligent Automation and 40% of companies have already deployed Generative AI solutions.
At Bitcot, we’re expanding our Automation and AI solutions with Gen AI to enable businesses to automate more complex processes.
Drop us a line and let’s explore how we can take your business to a whole new level.